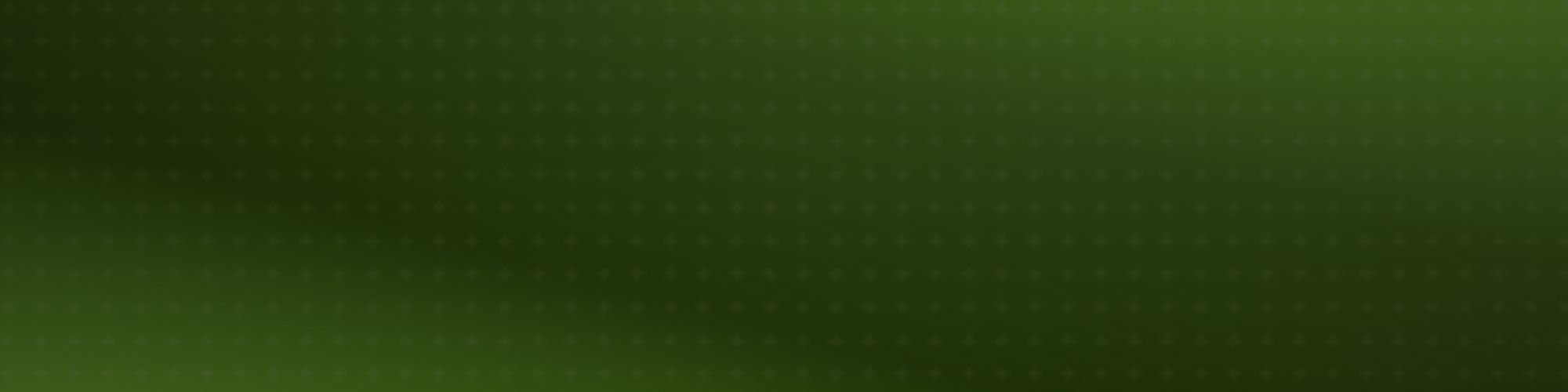
Revology Analytics Insider
Browse Contents Based on Category or Topic
Filter by Category
Filter by Topic/Tag
- AI
- AI Pricing
- AI in Business Analytics
- AI/ML
- AI/ML Distribution
- Analysis with Python
- Analysis with R
- Analysis with Tableau
- Analytics Leadership
- Attribution Modeling
- Bayesian Modeling
- Budget Optimization
- Business Growth through Pricing
- CPG
- CRM Data Analysis
- Commercial Analytics Transformation
- Competitive Analysis
- Competitive Pricing
- Consumer Durables
- Consumer Products
- Cross-Sell Optimization
- Customer Analytics
- Customer Churn Modeling
- Customer Retention
- Customer Segmentation
- Data Visualization
- Data Warehouse
- Data-Driven Decision Making
- Data-driven Pricing
- Discount Management
- Distribution
- Durable Goods
- Dynamic Pricing
- Forecasting
- Growth Drivers Analysis
- HiPPO effect
- In-sourced Pricing
- Industry Margin Pools
- Inventory
- Inventory Waste
- Knowledge Graphs
- Machine Learning
- Manufacturing
- Margin Analytics
- Margin Optimization
- Marketing Analytics
- Marketing Effectiveness & Optimization
- Marketing Mix Modeling
- Maximizing Profit with Pricing
- Med-Tech
Subscribe to Revology Analytics Insider
Want to stay abreast of the latest Revenue Growth Analytics thought leadership by Revology?
Revenue Growth Analytics and Sales: It's a Team Sport
Commercial Analytics in B2B environments is a team sport: you need to win the trust and credibility of the Sales Team to accelerate the impact of analytics and drive sustainable Pricing and Data ROI for your company.
Read this brief opinion piece on why listening to and collaborating with your Sales teams is not optional - it's essential.
Is too much discounting preventing you from meeting profitability targets?
Price leakage is a big problem for most Manufacturers and Distributors and can have a considerable adverse effect on their Operating Profits. One of the big reasons price leakage occurs is not having an adequate Margin Analytics Platform deployed and used across the organization. This means no effective measurement systems track discounts and rebates versus guidelines or strategies.
In this brief guide, we will discuss how to build a robust in-house solution, leveraging standard technologies you are familiar with. Most executives in charge of Pricing/Margin are hesitant to go down the margin analytics platform path, thinking it will take years and $ millions to implement. We will show you how to achieve 80-90% potential value realization using simple techniques and analytical methods.
The Unproductivity Problem with Your Inventory
Unproductive inventory often accounts for 20-30% of wholesale businesses' merchandise. For many, it's just the cost of doing business. But it doesn't have to be that way. Unproductive inventory ties up your cash, erodes your profitability, and puts you at the risk of not meeting debt obligations for your asset-backed loans. Automated markdown pricing is one of the most effective ways to address this problem, and simple analytical solutions (no "ML and AI) can often yield significant benefits. Learn more below how.
I welcome your comments or similar experiences.
CRM system hygiene a top data priority
Fixing our CRM data hygiene should be a top leadership priority to drive sales productivity and revenue growth. However, for many B2B environments particularly in Manufacturing and Wholesale, there’s often a big disconnect between strategy and execution. Companies spend a disproportionate time and investment on market research studies to understand their buyer archetypes and personas, only to stop at great Power Points, executive updates and cross-functional pontifications.
Meanwhile, the CRM systems are plagued by outdated and missing data and no value- or needs-based segmentation information, which lays the foundation for automated lead scoring or prescriptive capabilities like upsell, cross-sell or churn mitigation.
Now is the time to act and start treating our holistic CRM data with the attention and priority it deserves!
Marketing Mix Modeling is Here To Stay
Thanks to the demise of 3rd party digital IDs, our old friend Marketing Mix Modeling (MMM) is here to stay! Learn about MMM, how it's done, and how it can be highly impactful to you and your company.
An executive’s advice for data scientists (and leadership) – Part II.
In a previous article, I wrote about the three main structural challenges that data scientists and their organizations face when maximizing their career satisfaction and business impact (data ROI).
This edition of Revology Analytics Insider will dive deeper into the first impediment ("mismatch between data scientist aspirations and corporate reality"). We'll decompose why it exists and make concrete recommendations to the data science community and company leaders on how to best address it.
A Guide to Rate-Mix Modeling to Accelerate Margin Performance
Companies are in the business of making money, and most often they care about maximizing their Revenues, Gross profit or Operating income. One of the biggest challenges companies face is the ability to correctly and systematically diagnose and isolate the individual drivers of key business performance changes and build fast, surgical actions to increase profitability. This week I talk about the benefits of doing a proper price-cost-volume-mix analysis for your business, and get you started on building a production level application with analysis examples and explanations in Excel and R.
An executive’s career advice for data scientists - Part I.
Data science, AI and ML have been overhyped for at least the last decade, resulting in often unrealistic and misaligned expectations between data scientists and employers. Over the next couple of weeks, I shed light on the three major challenges data scientists typically encounter in their companies and provide concrete suggestions on how to tackle them for both personal and organizational success. Would love to hear from you about your experiences!
The Science (and Art) of Estimating Price Elasticities
Most of us are familiar with the term customer price sensitivity as an important concept especially for sales, marketing and revenue management teams. It helps us understand how price changes affect demand, profitability or market share of our products or services. This week, I will describe the most popular analytical methods that help you measure your product or service price elasticities, including a few simple and proven machine learning based approaches that your analytics or data science teams can easily do.
Monetize your Data with Operational Optimization
Last week I wrote about the key tenets for building analytics teams for real, measurable impact in your organization. This week, I’ll focus on one of the four fundamental #datamonetization strategies that companies should employ: capitalizing on their data assets to deploy #operational improvement initiatives that drive cost savings, revenue increases or both. Operational #optimization initiatives are usually a good place for companies to start their #analytics journey, assuming some foundational data capabilities are already in place: reliable internal data, decent #datagovernance and tech stack, a good understanding of customer behavioral profiles and foundational #datascience capabilities.
Read about key analytics use cases across three industries that optimize operational processes to drive real performance. If you have your own analytics use case stories from the trenches (successes or lessons learned), or just want to chat analytics, machine learning or revenue management, drop me a note.
Building analytics teams for real impact
Most analytics transformation efforts do not deliver a positive ROI for the enterprise even after several years. CEOs and their boards know they need to execute an AI-led differentiation strategy to either future-proof themselves, address an existential risk in the marketplace, or simply to make some operational improvements to their business. Yet, according to most estimates, 75-90% of digital transformations fail and less than 1 in 5 companies have fully extracted value out of their analytics journey. Most organizations fail on the last-mile delivery – in other words, front-line employees and decision-makers are not using the analytics tools and processes as intended, or not using at all.
Over the last decade of leading analytics teams, I have succeeded and failed many times. Below are five lessons learned from these experiences. I hope it can serve as a helpful 10K foot roadmap for nascent or aspiring analytics leaders or seasoned business executives who want to build a sustainable practice that adds real, quantifiable value for their company and its customers.